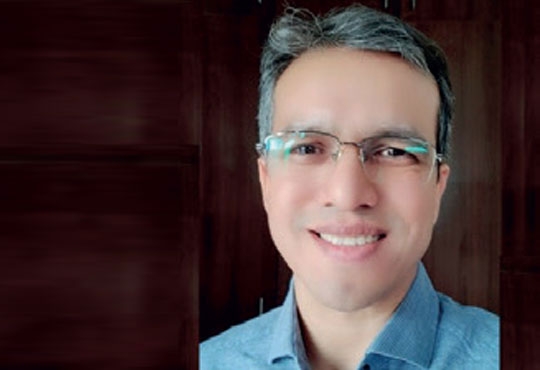
A 3-Pronged Framework To Ensure Smooth Artificial Intelligence(AI) Adoption In Enterprises
Deepak Arora, VP - Technology, Publicis Sapient | Tuesday, 15 February 2022, 07:37 IST
Offering an entire gamut of capabilities to solve complex business problems, Machine Learning (ML) and Artificial Intelligence (AI) are imperatives for business success today. A recent survey reveals 85 percent of businesses are either evaluating AI or already using some basic form of it in production. Most businesses face the same set of challenges while embracing AI: Lack of a strategic view and alignment of needs. Recognizing and establishing use cases. Shortage of skills in the market...
How should organizations go about addressing these bottlenecks? How can business and tech leaders adopt AI to its true potential and drive value for the enterprise?
Most of these bottlenecks can be mapped to three categories:
Strategy
Business and tech leaders should take a more scientific approach to addressing the two most important challenges: 1. Recognizing the need for AI and 2. Difficulties in identifying use cases.
·Explore ‘Prediction' opportunity: To recognize the need for AI and establishing use cases leaders should review organizational workflows and break them into tasks. They must analyze the tasks that have a significant prediction component and would benefit from a prediction machine. Next, they should determine the ROI for building a prediction machine to complete each task, and rank those tasks from top to bottom. The next step is to prioritize the tasks and adopt a POV on how fast they want to reach the transformation point so they can determine the necessary investment.
· Define clear goals at use case level: According to McKinsey, "The organizations that will benefit most from AI will be the ones that are able to most clearly and accurately specify their objectives." In my opinion, clear objectives will not only help in understanding transformational potential, but will also help define data and training requirements. Thanks to the methods used to train AI, its effectiveness is directly tied to goal specification clarity.
"The 3x3 charter is a blueprint for enterprises to scale and realize the true potential of their AI initiatives"
Platform
For many organizations, deriving value from AI/ML is an expensive proposition. This is further compounded in large companies where multiple teams are eager to adopt AI/ML. However, in most cases:
·Different teams use different methods to approach these initiatives.
·Those experimenting are doing so at a premium: procuring infrastructure, creating data connectors and pipelines, and deploying manually.
Too much energy is spent on activities that do not produce value, which further contributes to adoption barriers.
·Implement ‘As a Service' pattern: In ML, data is collected, unified, cleaned and then stored for analysis and training of new models. The ML workspace is used for the experimentation, creation and training of models. Once the desired results are achieved, the models are made available by publishing them as a web service. This model caters to diverse use cases, supporting varied sets of data sources. The activation can be done differently for different teams, thereby doing justice to the `As a Service' pattern and reducing adoption issues.
·Leverage Cloud platform capabilities and avoid re-inventing the wheel: All leading cloud providers provide great solutions to realise the true potential of AI ‘As a Service' pattern. Below is an indicative view of how this can be explored across the leading cloud providers:
·Strive for a ‘No-Code' workspace: No-code ML model workspaces are guided platforms that allow users to drag and drop their data pipelines and models to test. Guidelines for how to productionize and scale models beyond a single domain help users across different teams where specific skills may be missing, thereby further reducing adoption barriers.
Talent
‘As a Service' deployments and adoption of cloud help business and tech leaders significantly address their talent challenges. However, I think there's still more work to be done, especially since Data Science and Data Engineering could easily top the skill gap charts across organizations.
·Great ‘Bespoke' as exception: Data processing tools and technologies have evolved phenomenally over the last five years. Enterprises should first explore the various off the shelf options that are available, before they decide to build them themselves.
·Be selective: Leaders should identify the work they need their Data Scientists and Engineers to do. Engineers should be leveraged to optimize core data infrastructure, or build tools when there's no off-the-shelf capability available. Similarly, Data Scientists should be deployed for statistics, probability, mathematics and algorithms, and not for coding.
·Upskill to address gaps: Data Engineering skills can be learned relatively easily (compared to Data Science skills), and organizations should invest in addressing that gap internally.
Summing it up
The 3x3 charter is a blueprint for enterprises to scale and realize the true potential of their AI initiatives. It can guide them through their AI adoption journey. The ideal way: start with ‘Strategy', and then go through each stage, evolving the roadmap as their business and AI itself transforms.
CIO Viewpoint
Data Science & Machine Learning In Fintech
By Jainendra Kumar, Head - Global Delivery Center-India & Senior Director Product Development Software, Diebold NixDorf
The Art & Science of Insight-Driven Modern-Day...
By By Preeti Das, Global head- IT & Digital Services, Sutherland Global Services
CXO Insights
How Low-Code Is Transforming The Future Of Work...
By Deepak Pargaonkar, VP - Solution Engineering, Salesforce
3 Focus Areas For An Autonomous Driving Revolution
By Varun Chhabra, Vice President, Product Marketing Cloud, Dell Technologies
A 3-Pronged Framework To Ensure Smooth...