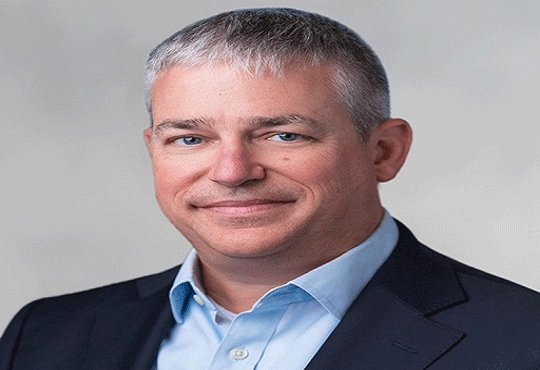
Top Three Use-cases of Synthetic dataset
Abhrasnata Das | Thursday, 05 January 2023, 17:55 IST
Artificial intelligence (AI) is now finding increased use in business functions including insurance and financial services. Businesses trying to preserve market supremacy are using AI technology more often, and 2023 will be a year of fast growth. One of the most important things C-level executives need to grasp is why AI is the specific instrument they need to produce AI.
Companies may unlock the value of sensitive client data by using tools like synthetic data generators powered by cutting-edge AI engines, while still protecting customer privacy and abiding by data protection rules.
By 2024, 60% of the data required to construct AI and analytics projects will be artificially generated, predicts Gartner. Without synthetic data generation, responsible AI in particular cannot exist. Datasets containing biases can be rebalanced throughout the synthesis process. The final product is synthetic data that is completely privacy-compliant and acceptable for giving explainability to people developing AI and examining the model for compliance and ethics.
In this article we have listed three key areas where Synthetic dataset will be of vital importance.
Granular Data Sharing in Healthcare and Pharma
Health care depends on data access and analysis for research and innovation more than maybe any other industry. It is crucial to have the capacity to use precise, statistically accurate data.
Healthcare and pharmaceuticals are among the most heavily regulated sectors, and they must also deal with challenging concerns including data access, patient privacy, and reducing the likelihood of data breaches and information silos.
Synthetic data are completely anonymous and do not need further patient consent, which opens up a wide range of new opportunities. The adaptability of this data format can spur creativity and assist businesses in fully grasping patients and ailments.
Synthetic data analysis, for instance, can help with quicker illness or medication development, a more individualized approach to patient care, and better patient outcomes.
AI-Driven Data Agility in Insurance
Insurance firms must make the most of the data they gather due to evolving business processes, new customer demands, and fierce competition. Regulations governing data security and privacy, as well as outdated and compartmentalized systems, limit their capacity to do so.
Privacy-preserving synthetic data may be able to assist insurers in overcoming some of their data processing difficulties as legislation and consumer expectations for data privacy and security continue to climb.
In the insurance industry, it is possible to synthesize the majority of tabular customer data, including claim data, sales and attrition data, as well as market and survey data. Using data that conforms with the strongest privacy and regulatory standards, it is possible to discover, create, and test new products that address client demands.
For instance, the customer experience may be enhanced to boost conversion rates by providing real-time and secure information flow between departments and jurisdictions.
Enhanced Analytics in Telco
The telecom sector also faces constraints related to data retention. Most laws stipulate that data must be erased after a certain amount of time. As a result, creating an analysis that spans a longer time frame is nearly never feasible.
For instance, a business would want access to at least two years' worth of data in order to comprehend seasonality. A data retention policy makes it challenging to comprehend customer attrition or customer migration, or to evaluate the long-term effects of choices.
In this situation, using learnings from the original data, synthetic data may be utilized to build a pipeline of synthetic customers. With synthetic client data, the original data patterns will be kept without keeping any particulars about the consumer. Without keeping the original data, a corporation may utilize this data for practically all types of analytics.
Better than Real Data?
Compared to real-world data, synthetic data is far more scalable and intelligent. But it will take more work than employing an AI tool to create reliable synthetic data. Companies are now required to have a solid understanding of AI and specific expertise of handling different frameworks if they want to produce accurate and reliable synthetic data.
Additionally, the dataset shouldn't contain any trained models that might distort it and make it unrepresentative of reality. By providing an accurate depiction of the real-world data and taking into account the current biases, this will correct the datasets. Using this technique, one may achieve their objectives and produce synthetic data.
It is well-known that synthetic data aims at facilitating data scientists on accomplishing new and innovative things which will be tougher to achieve with real-world data, so we can surely assume that synthetic data is the future.
CIO Viewpoint
India and its Data Center Advancements
By By Michael Cantor, CIO, Park Place Technologies
How AI/Machine Learning can Revamp Data Centers...
By Piyush Kumar Chowhan, CIO and Vice President, Arvind Lifestyle Brands Limited
By Sanjay Chowdhry, CIO, Hamdard WAKF Laboratories
CXO Insights
Why A Data First Approach Could Be Your...
By Geetha Ramamoorthi, Managing Director, India, KBR Inc
A Short Guide for Data-driven and...
By Kapil Makhija, VP - Technology Cloud Business, Oracle India
The burgeoning market of prompt engineering in...