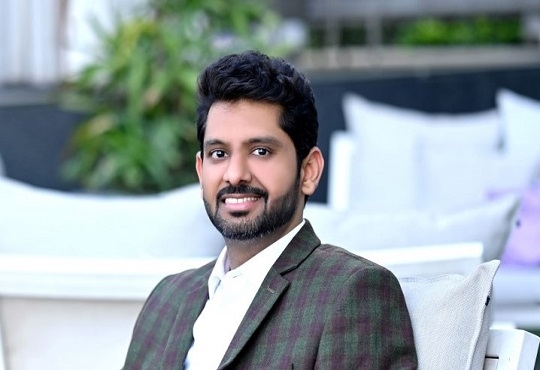
AI Integration in Multi-Cloud and Edge Environments
FaizShakir, VP & Managing Director – Sales, Nutanix | Tuesday, 19 December 2023, 10:28 IST
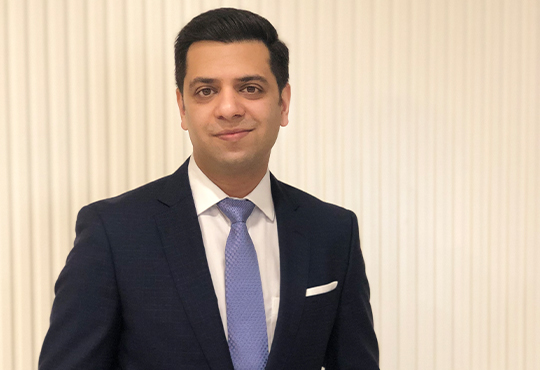
In an interaction with CIOtechoutlook magazine, Faiz discusses AI integration benefits, tools interoperability, deployment challenges, edge computing's impact on decision-making, and emerging strategies for managing AI workloads across cloud environments.
How are organizations effectively integrating AI capabilities into their multi-cloud and edge computing strategies to gain a competitive edge?
Organizations are effectively integrating AI capabilities into their multi-cloud and edge computing strategies to gain a competitive edge in several ways. Adopting a hybrid cloud approach allows organizations to combine the benefits of public and private clouds, enabling them to optimize AI performance and scalability. While edge computing brings AI processing closer to the source of data, enabling real-time decision-making and latency-sensitive applications. For example, edge computing can be used for real-time fraud detection.
What role does the interoperability of AI tools and frameworks play in ensuring seamless AI integration across private clouds, public clouds, and edge environments?
According to the Nutanix State of Enterprise AI Report, 86% of APJ respondents plan to purchase existing AI models or leverage existing open-source AI models in order to build their AI applications, which is similar to the global average. The interoperability of AI tools and frameworks plays a crucial role in ensuring seamless AI integration across private clouds, public clouds, and edge environments. Without interoperability, organizations would face significant challenges in moving AI models and workloads between different environments, to achieve interoperability, several standards and initiatives are being developed by industry leaders and open-source communities.
For example:
Open Neural Network Exchange (ONNX) is an open format for representing AI models, allowing them to be easily exchanged between different frameworks.
MLI is a set of specifications for interoperability between machine learning frameworks, including TensorFlow, PyTorch, and MXNet.
By adopting such initiatives, companies can ensure that their AI tools and frameworks are interoperable which would allow them to seamlessly integrate AI across their multi-cloud and edge computing environments.
What are the primary challenges and considerations when deploying AI models in a distributed computing landscape that spans private, public, and edge clouds?
Deploying AI models in a distributed computing landscape that spans over private, public, and edge clouds presents several challenges and considerations that organizations must address to ensure successful implementation. These challenges include:
Data management and security
Distributed AI deployments require effective data management strategies to ensure data privacy, security, and compliance across different cloud environments. Organizations need to establish clear data governance policies and implement robust security measures to protect sensitive data.
Model management and versioning
Managing AI models across multiple environments can be complex, especially when models evolve over time. Organizations need to implement model management tools and versioning systems to track changes, maintain consistency, and ensure that the right version of the model is deployed in the correct environment.Organizations can effectively implement AI models in a distributed computing environment and enjoy increased productivity, better decision-making, and cutting-edge goods and services by carefully addressing such challenges.
How does the combination of edge computing and AI impact real-time data processing and decision-making in organizations with dynamic workloads and data distribution requirements?
The combination of edge computing and AI has a transformative impact on real-time data processing and decision-making in organizations with dynamic workloads and data distribution requirements. Edge computing enables real-time data processing and analysis, significantly reducing the time it takes to extract insights and make decisions from data. By processing data locally, organizations can respond to events and anomalies in real time, enabling proactive measures and preventing potential disruptions. This is essential for applications where immediate action is required, such as fraud detection, network traffic management, and predictive maintenance. Edge computing also helps in reducing the amount of data that needs to be transmitted to centralized cloud servers, minimizing network bandwidth usage and associated costs. This can be particularly beneficial for organizations with large volumes of data generated at remote locations.
What strategies and best practices are emerging for managing AI workloads and data across multiple cloud environments while optimizing performance and cost-efficiency?
Emerging strategies for managing AI workloads and data across multiple cloud environments are underscored by compelling statistics. The report reveals that 90% of organizations consider AI a priority, with 84% planning to modernize their IT infrastructure and 83% aiming to advance their edge strategy. Data security and governance take precedence, with over 90% of respondents emphasizing considerations such as data security, quality, scalability, and speed of development in running their AI workloads. Furthermore, all surveyed organizations express the need for additional AI skills, highlighting a skills shortage in AI modeling and application development. The report indicates that 99% of respondents plan to upgrade their AI applications or infrastructure, with more than half focusing on improving data transfer between cloud, data center, and edge environments. These statistics reflect a concerted effort to align AI strategies with data governance, infrastructure modernization, and skill development to optimize performance and cost-efficiency in the rapidly evolving landscape of enterprise AI adoption.
CIO Viewpoint
Unlocking the Potential of Cloud and AI: A...
By Pratik Jain, Lead Business Analyst – Digital Transformation, ACS Global Tech Solutions
Importance of Zero-Trust Cloud Security in the...
By Sameer Danave, Senior Director Marketing, MSys Technologies
The Transition to a Cloud-First World
By Kapil Makhija, Vice President -Technology Cloud, Oracle India
CXO Insights
Balancing Generative AI Capabilities with Data...
By Murad Wagh, Director - Sales Engineering, Snowflake
AI Integration in Multi-Cloud and Edge...
By FaizShakir, VP & Managing Director – Sales, Nutanix
The Role of Hybrid Cloud in ERP Integration