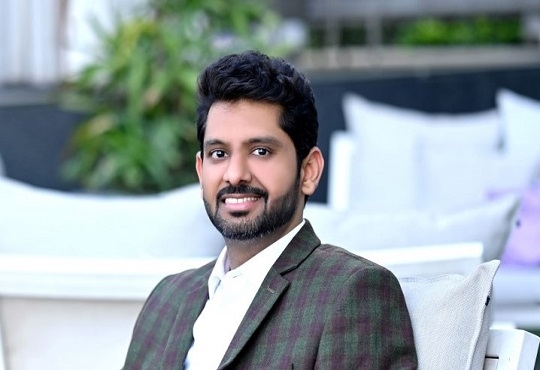
Balancing Generative AI Capabilities with Data Privacy: Strategies and Challenges
Murad Wagh, Director - Sales Engineering, Snowflake | Thursday, 25 April 2024, 12:51 IST
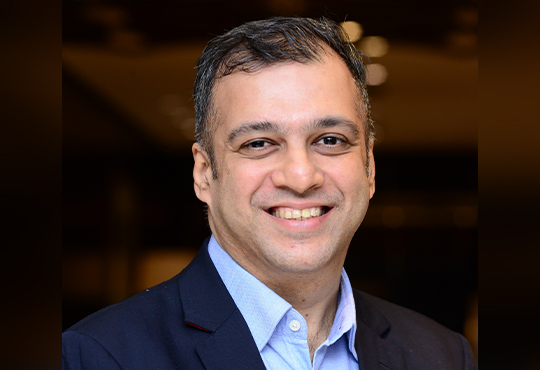
In a conversation with CIOTechOutlook Magazine, Murad Wagh shared his views and thoughts on how companies can streamline the process of securely leveraging generative AI capabilities while ensuring compliance with data privacy regulations, as well as the main challenges organizations face in implementing generative AI and large language models while maintaining data privacy.
How does generative AI impact data privacy, and what steps do you recommend for managing these concerns?
There are significant data privacy implications that must be considered when training large language models and harnessing generative AI — for both commercial and non-commercial use. At Snowflake, data governance is of the utmost importance. We’ve engineered a platform that consolidates data into a single repository, establishing a definitive truth and integrating a governance framework. Our approach to governance begins with identification, distinguishing critical data requiring protection, such as Personally Identifiable Information (PII). Not all organizational data necessitates the same level of protection, so our platform offers diverse capabilities like tokenization, masking, and role-based access control for safeguarding. Finally, ensuring the consistent application of these policies across all data access points remains paramount. This steadfast commitment to governance underscores our platform's integrity, ensuring robust data protection and privacy measures are upheld.
What strategies would you recommend for safeguarding sensitive data within cloud storage environments like data warehouse, Data Lake, and hybrid architectures?
The key to safeguarding sensitive data within cloud storage environments lies in adopting a multi-layered security and governance approach. Laying out a policy that defines data classification, tagging and policy based masking is critical. Implementing robust encryption protocols for data at rest and in transit is imperative. Additionally, access controls should be tightly managed, ensuring that only authorized users have access to sensitive data. Regular security audits and monitoring, coupled with advanced threat detection mechanisms, are also essential to identify and mitigate potential vulnerabilities or breaches promptly.
How can companies streamline the process of securely leveraging generative AI capabilities while ensuring compliance with data privacy regulations?
As companies strive to leverage generative AI capabilities securely and comply with data privacy regulations, it's crucial to establish a robust data strategy and governance framework. By integrating security features like encryption and access controls, companies can protect sensitive data while enabling seamless collaboration. We secure Data Sharing facilitates direct access to live data without additional integration work, preserving privacy. By prioritizing security and compliance in data sharing practices, companies can streamline the process of leveraging generative AI while safeguarding data privacy and meeting regulatory requirements.
What are the main challenges organizations face in implementing generative AI and Large Language Models (LLMs) while maintaining data privacy?
When tackling the challenges of implementing generative AI and LLMs, it's crucial to recognize the differing needs of consumers and enterprises. While consumers enjoy flexibility, enterprises must prioritize building robust foundations for AI and related data strategies, given their responsibility to protect customer data and intellectual property. As businesses adopt transformative technologies like AI, risk assessment becomes paramount, with data integrity at the forefront. Hence, establishing a strong data strategy forms the bedrock for successful AI implementation. Consolidating data into a single source of truth poses a significant challenge for many companies, but we supports organizations in this critical step. Our unique security approach keeps the data within the organization's security perimeter while allowing models to operate securely.
What proactive measures should companies take to address data privacy challenges associated with emerging technologies like generative AI?
By 2025, Gartner expects generative AI to account for 10% of all data generated. When LLMs lack privacy-preservation during training, they may become susceptible to various privacy threats and attacks. As companies venture into realms like generative AI, it's paramount to take proactive measures to effectively address data privacy challenges. Responsible development and deployment of AI are imperative, ensuring transparent and secure data collection and processing while empowering individuals with data control. Moreover, AI systems necessitate meticulous design, testing, and continuous monitoring to identify and rectify biases.
How do robust architecture and frameworks contribute to enhancing data privacy when deploying LLMs?
When it comes to enhancing data privacy with LLMs, having a solid architecture and frameworks in place is crucial. These systems let organizations categorize their data based on its sensitivity, so they can implement specific security measures. With granular access controls, only authorized users can access LLMs, while techniques like anonymization and masking safeguard sensitive data. By integrating compliance frameworks, organizations can adhere to data privacy regulations, ensuring they follow the necessary privacy-enhancing practices. We provide a range of capabilities, including tokenization, masking, and role-based access control, to manage data according to its sensitivity levels. This helps control access with precise policies and supports anonymization and masking for protecting sensitive data while enabling analysis.
CIO Viewpoint
Unlocking the Potential of Cloud and AI: A...
By Pratik Jain, Lead Business Analyst – Digital Transformation, ACS Global Tech Solutions
Importance of Zero-Trust Cloud Security in the...
By Sameer Danave, Senior Director Marketing, MSys Technologies
The Transition to a Cloud-First World
By Kapil Makhija, Vice President -Technology Cloud, Oracle India
CXO Insights
Balancing Generative AI Capabilities with Data...
By Murad Wagh, Director - Sales Engineering, Snowflake
AI Integration in Multi-Cloud and Edge...
By FaizShakir, VP & Managing Director – Sales, Nutanix
The Role of Hybrid Cloud in ERP Integration